Figure 1. Delineation of the epileptogenic zone by using AI-based tools. (A) Multimodal data acquired before and after the resection operation is preprocessed to extract the channel coordinates, the resection volume, and the clinically defined SOZ. (b) iEEG time series data is preprocessed to clean and filter the data. Different dynamic features are extracted from the signals and processed using unsupervised and supervised machine learning algorithms to identify epileptic networks from interictal and ictal data. The resulting networks are analyzed statistically against gold standards and evaluated for outcome prediction.
Artificial Intelligence in Epilepsy
Artificial Intelligence (AI) refers to the field of computer science and technology that focuses on creating systems and software capable of performing tasks that typically require human intelligence. These tasks include problem-solving, decision-making, learning, reasoning, and automatically analyzing complex multimodal data. AI has revolutionized health care by providing advanced tools and technologies that enhance diagnostic accuracy, treatment efficiency, and patient care.
Making use of the advances in AI, our team is currently working on developing AI-based automated tools to develop epilepsy biomarkers that accurately delineate the epileptogenic zone in children with drug resistant epilepsy. By using signal decomposition methods, machine learning algorithms, and brain modeling techniques, our team aims to develop complex multimodal data analysis tools that incorporate information from multiple imaging modalities and help epileptologists improve surgical planning for patients with drug resistant epilepsy.
The team is currently studying dominant patterns in interictal and ictal intracranial electroencephalography (EEG) data in different physiological frequency bands. The aim is to automatically identify epileptic brain regions and study their ability to predict surgical outcome (Fig. 1).
In the future, diverse brain imaging modalities will be used simultaneously to model the dynamics of the brain during seizures by constructing patient-specific digital twins using AI that may help enhance the evaluation, diagnosis, and prognosis of children with drug resistant epilepsy.
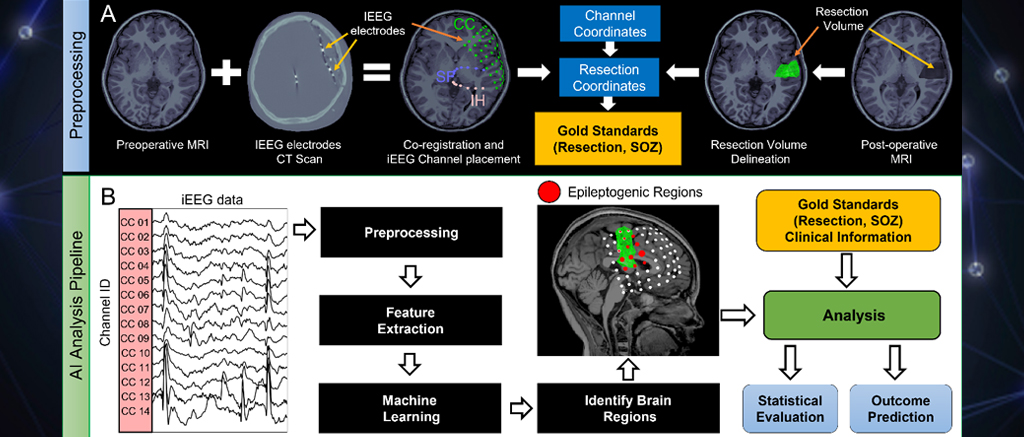